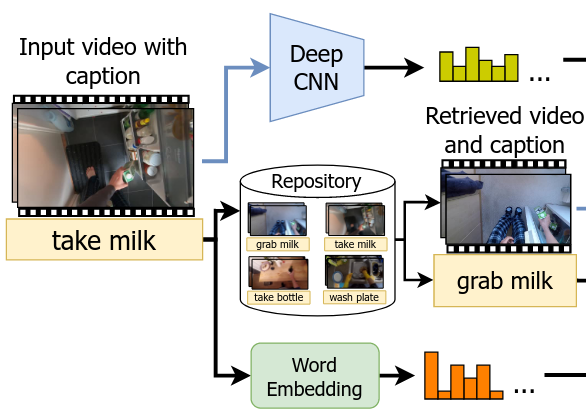
The text-to-video retrieval task requires to rank all the videos in a database based on how semantically close they are to an input query. To do so, both the visual and the textual contents need to be carefully analyzed and understood, meaning that a wide range of Computer Vision and Natural Language Processing techniques are required. Despite the intrinsic difficulty of such a problem, it is a fundamental one: in fact, nowadays several hundreds of hours of video content are uploaded to the Internet every minute, therefore solutions to this important problem are fundamental to perform searches effectively and retrieve all the videos which the user is looking for. Moreover, considering the need for multi-modal content understanding, advancements in this field may lead to improvements in many other problems, including Captioning and Question Answering.
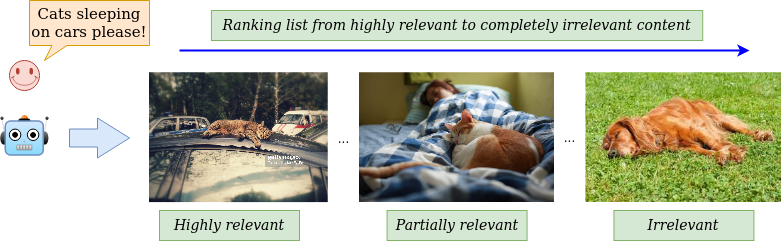
EPIC-Kitchens-100 Multi-Instance Retrieval Challenge tech reports:
- Alex Falcon, Giuseppe Serra. UniUD Submission to the EPIC-Kitchens-100 Multi-Instance Retrieval Challenge 2023. We ranked 3rd using only 25% of the training data! [report pdf]
- Alex Falcon, Giuseppe Serra, Sergio Escalera, Oswald Lanz. UniUD-FBK-UB-UniBZ Submission to the EPIC-Kitchens-100 Multi-Instance Retrieval Challenge 2022. We ranked π 1st! π [report pdf]
Related publications:
- Alex Falcon, Giuseppe Serra, Oswald Lanz. Improving semantic video retrieval models by training with a relevance-aware online mining strategy. Computer Vision and Image Understanding 245, 104035, August 2024. [pdf] [DOI]
- Alex Falcon, Giuseppe Serra, Oswald Lanz. A Feature-space Multimodal Data Augmentation Technique for Text-video Retrieval. ACM International Conference on Multimedia (ACM MM), 2022. [pdf] [DOI]
- Alex Falcon, Swathikiran Sudhakaran, Giuseppe Serra, Sergio Escalera, Oswald Lanz. Relevance-based Margin for Contrastively-trained Video Retrieval Models. ACM International Conference on Multimedia Retrieval (ICMR), 2022. [pdf] [DOI]
- Alex Falcon, Giuseppe Serra, Oswald Lanz. Learning Video Retrieval Models with Relevance-Aware Online Mining. International Conference on Image Analysis and Processing (ICIAP), 2021. [pdf] [DOI]
Research group:
- Alex Falcon (AILAB-Udine Member)
- Oswald Lanz (TeV β FBK, AILAB-Udine External Collaborator)
- Giuseppe Serra (AILAB-Udine member)