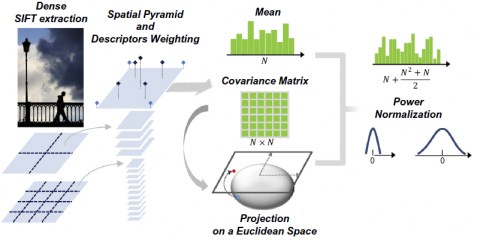
The Bag of Words paradigm has been the baseline from which several successful image classification solutions were developed in the last decade. These represent images by quantizing local descriptors and summarizing their distribution. The quantization step introduces a dependency on the dataset, that even if in some contexts significantly boosts the performance, severely limits its generalization capabilities. Differently, in this paper, we propose to model the local features distribution with a multivariate Gaussian, without any quantization.
The full rank covariance matrix, which lies on a Riemannian manifold, is projected on the tangent Euclidean space and concatenated to the mean vector. The resulting representation, a Gaussian of Local Descriptors (GOLD), allows to use the dot product to closely approximate a distance between distributions without the need for expensive kernel computations. We describe an image by an improved spatial pyramid, which avoids boundary effects with soft assignment: local descriptors contribute to neighboring Gaussians, forming a weighted spatial pyramid of GOLD descriptors. In addition, we extend the model leveraging dataset characteristics in a mixture of Gaussian formulation further improving the classification accuracy. To deal with large scale datasets and high dimensional feature spaces the Stochastic Gradient Descent solver is adopted. Experimental results on several publicly available datasets show that the proposed method obtains state-of-the-art performance.
The Research Group achieved the Best Performance of the International IMAGECLEF Competition on the Imageretrieval task; about 40 research groups took part in the Competition.
Related publications:
- Serra G, Grana C, Manfredi M, Cucchiara R. GOLD: Gaussians of Local Descriptors for image representation. Computer Vision and Image Understanding. 2015;134:22-32.
- Serra G, Grana C, Manfredi M, Cucchiara R. Covariance of Covariance Features for Image Classification. In: Proc. of the ACM International Conference on Multimedia Retrieval. Glasgow, Scotland; 2014.
- Serra G, Grana C, Manfredi M, Cucchiara R. Modeling Local Descriptors with Multivariate Gaussians for Object and Scene Recognition. In: Proc. of ACM International Conference on Multimedia (MM). Barcelona, Spain; 2013.
- Grana C, Serra G, Manfredi M, Cucchiara R. Beyond Bag of Words for Concept Detection and Search of Cultural Heritage Archives. In: Proc. of International Conference on Similarity Search and Applications (SISAP). A CoruΓ±a, Spain; 2013
- Grana C, Serra G, Manfredi M, Cucchiara R, Mandreoli F, Martoglia R. UNIMORE at ImageCLEF 2013: Scalable Concept Image Annotation. In: CLEF 2013 Working Notes. Valencia, Spain; 2013.
- Grana C, Serra G, Manfredi M, Cucchiara R. Image Classification with Multivariate Gaussian Descriptors. In: Proc. of International Conference on Image Analysis and Processing (ICIAP). Napoli, Italy; 2013.
Resources
We release the MATLAB implementation of the copy-move detection approach presented in Serra et al., CVIU 2015.
GOLD β released May 8, 2012 (tested on Linux Ubuntu 10.04) If you use our software or these datasets, please cite the paper: Serra G, Grana C, Manfredi M, Cucchiara R. GOLD: Gaussians of Local Descriptors for image representation. Computer Vision and Image Understanding. 2015;134:22-32.