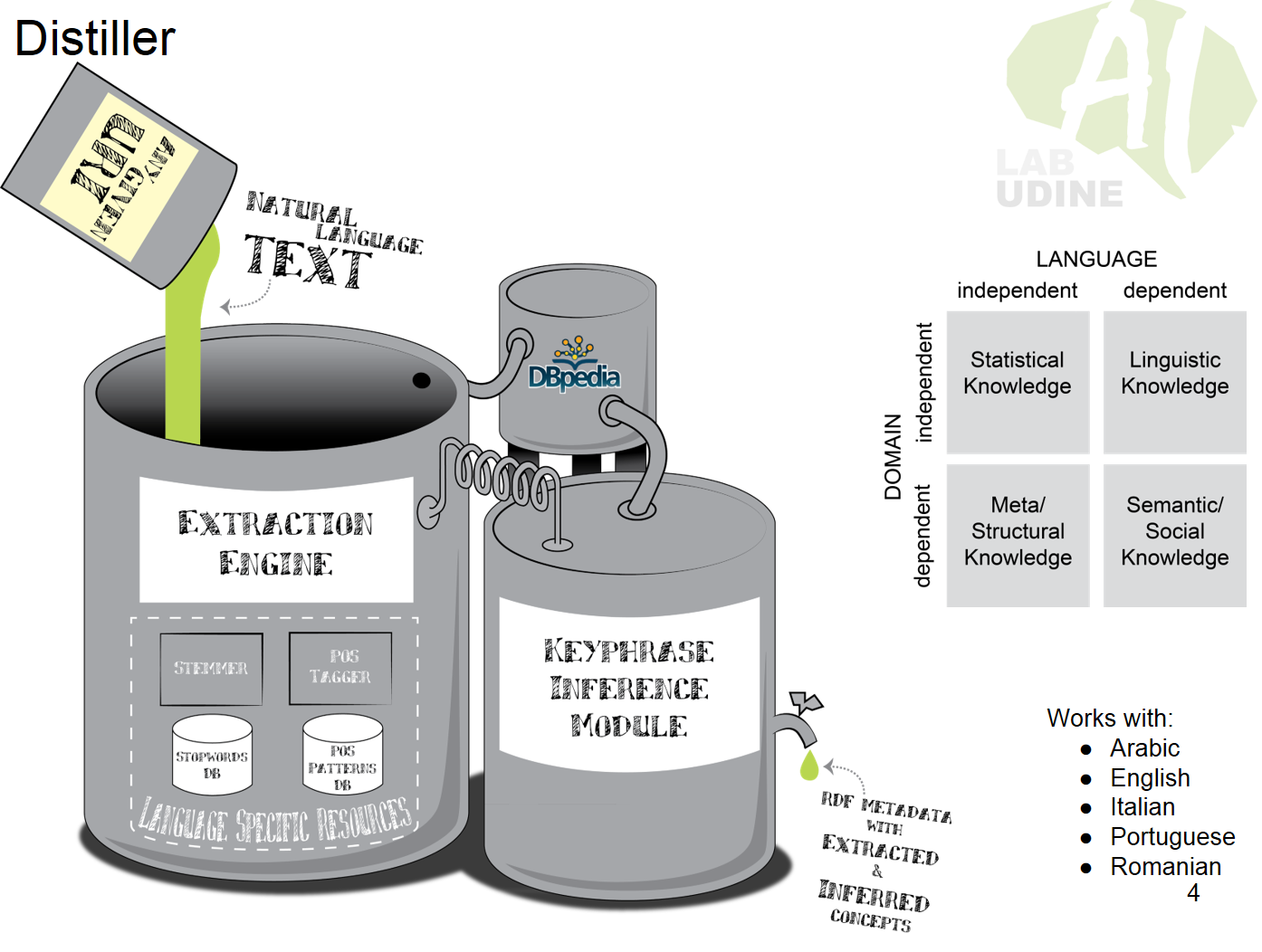
Keyphrases (KPs) are phrases that “capture the main topic discussed on a given document”. More specifically, KPs are phrases typically one to five words long that appear verbatim in a document, and can be used to briefly summarize its content. The task of finding such KPs is called Automatic Keyphrase Extraction (AKE). Recently, AKE has received a lot of attention, because it has been successfully used in many natural language processing (NLP) tasks, such as text summarization, document clustering, or non-NLP tasks such as social network analysis or user modeling. AKE approaches have been also applied in Information Retrieval of relevant documents in digital document archives which can contain heterogeneous types of items, such as books articles, papers etc.
However, given the wide variety of lexical, linguistic and semantic aspects that can contribute to define a keyphrase, it difficult to design hand-crafted feature, and even the best performing algorithms hardly reach F1-Scores of 50% on the most common evaluation sets. For this reason, AKE is still far from being a solved problem in the NLP community.
Distiller is a framework, whose main aim is to support research and prototyping activities by providing an environment for building testbed systems and integrating existing AKE systems. The design of Distiller is guided by the key principle that several different types of knowledge are involved in the process of AKE and should be clearly separated in order to design systems able to cope with multilinguality and multidomain issues. Distiller is organized in a series of single-knowledge oriented modules, where any module is designed to perform a single task efficiently, e.g. language processing, statistical analysis, knowledge inference, and so on. This allows a highly modular design with the possibility of implementing different pipelines (i.e. sequences of modules) for different tasks. Distiller supports five languages including English, Arabic, Italian, Portuguese, and Romanian.
Test our Demo!!!
Related publications:
- Basaldella M, Antolli E, Serra G, Tasso C., Bidirectional LSTM Recurrent Neural Networkfor Keyphrase Extraction, Italian Research Conference on Digital Libraries (IRCDL), 2018.