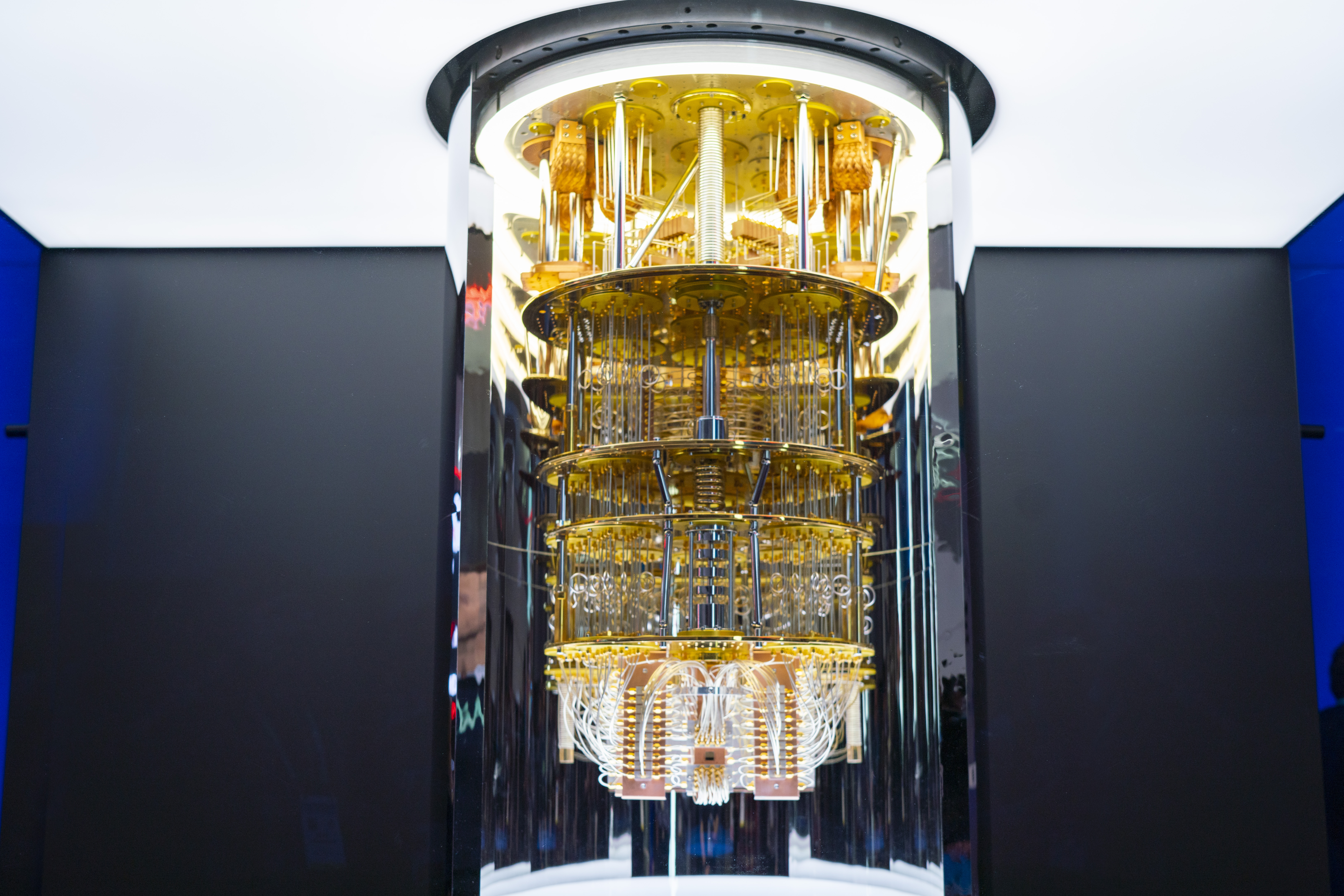
Quantum machine learning (QML) is an emerging field that combines the principles of quantum computing with classical machine learning techniques. It aims to exploit the unique properties of quantum systems, characterised by their ability to perform computations exponentially faster than classical devices, to improve the processing and analysis of complex data. This advance makes QML a very promising frontier. However, in the current Noisy Intermediate-Scale Quantum (NISQ) era, characterised by the presence of noise in quantum devices that limits their scalability, it is crucial to develop specific techniques to fully exploit quantum capabilities.
This research focuses on the development and optimisation of QML models suitable for NISQ environments. A key aspect of our work is to adapt established optimisation methods from classical machine learning, such as batch normalisation and regularisation, for use in Quantum Neural Networks (QNNs). The project also explores the potential of Quanvolutional Neural Networks and Quantum Kernel methods. These efforts are aimed at improving the functionality and efficiency of QML systems in dealing with the limitations of current quantum hardware.
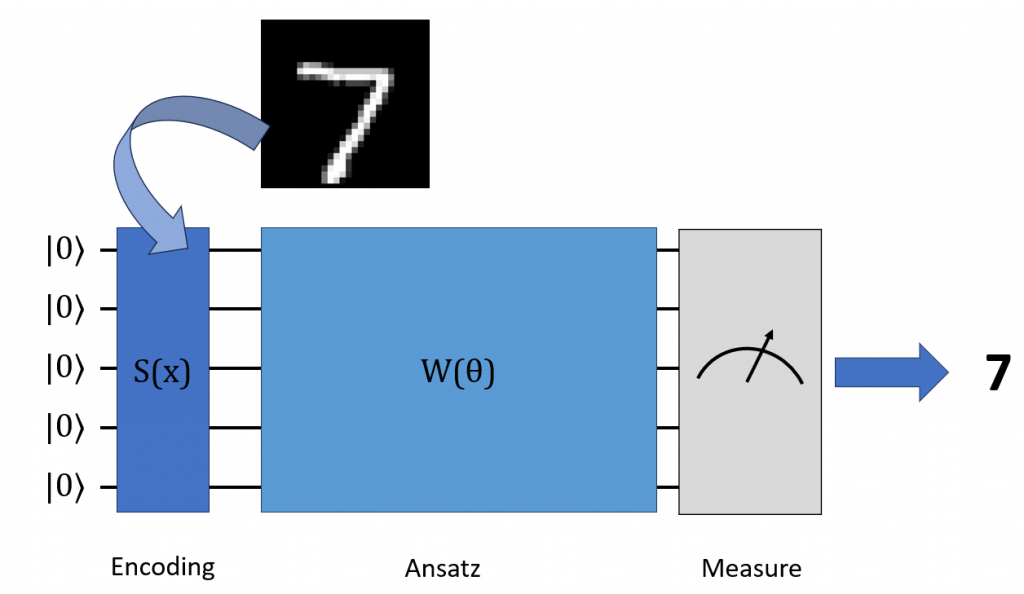
Related publications:
- Massimiliano Incudini, Daniele Lizzio Bosco, Francesco Martini, Michele Grossi, Giuseppe Serra, Alessandra Di Pierro. Automatic and effective discovery of quantum kernels. preprint, 2024.
Research group:
- Daniele Lizzio Bosco (AILAB-Udine Member)
- Beatrice Portelli (AILAB-Udine Member)
- Giuseppe Serra (AILAB-Udine Member)