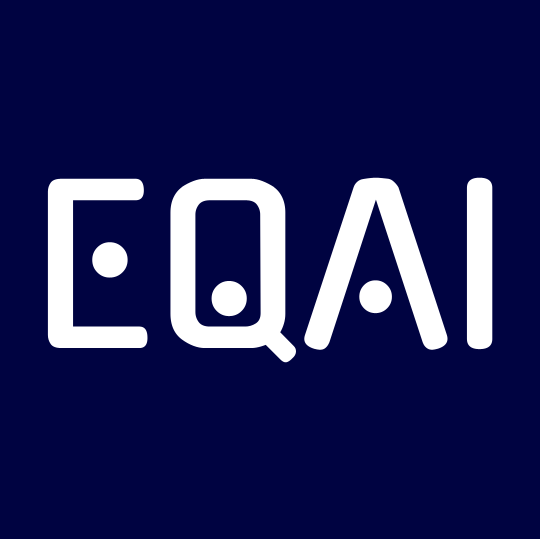
AILAB-Udine is proud to be one of the organizers of the European Summer School on Quantum AI, (EQAI), which has been held yearly since 2022. Find the latest updated information on the official website:http://eqai.eu Or subscribe to the official google group to be notified of any major update!https://groups.google.com/g/eqai The summer school will take place in Lignano Sabbiadoro (Italy) on September 01 – 05, 2025.All the speakers will be there in person to make the experience more immersive and interactive! Find out more about the past editions at: https://eqai.eu/past-editions/ EQAI 2024 – Details The summer school will take place in Lignano Sabbiadoro (Italy) on September 02 – 06, 2024. EQAI 2023 – Details The summer school will take place in Udine (Italy) on May 29 – June 1, 2023, but it can also be followed remotely. The main topic of this edition is “Quantum Machine and Deep Learning”. Deadlines for application are: ▶ on-site: April 29, 2023, ▶ remote: May 15, […]