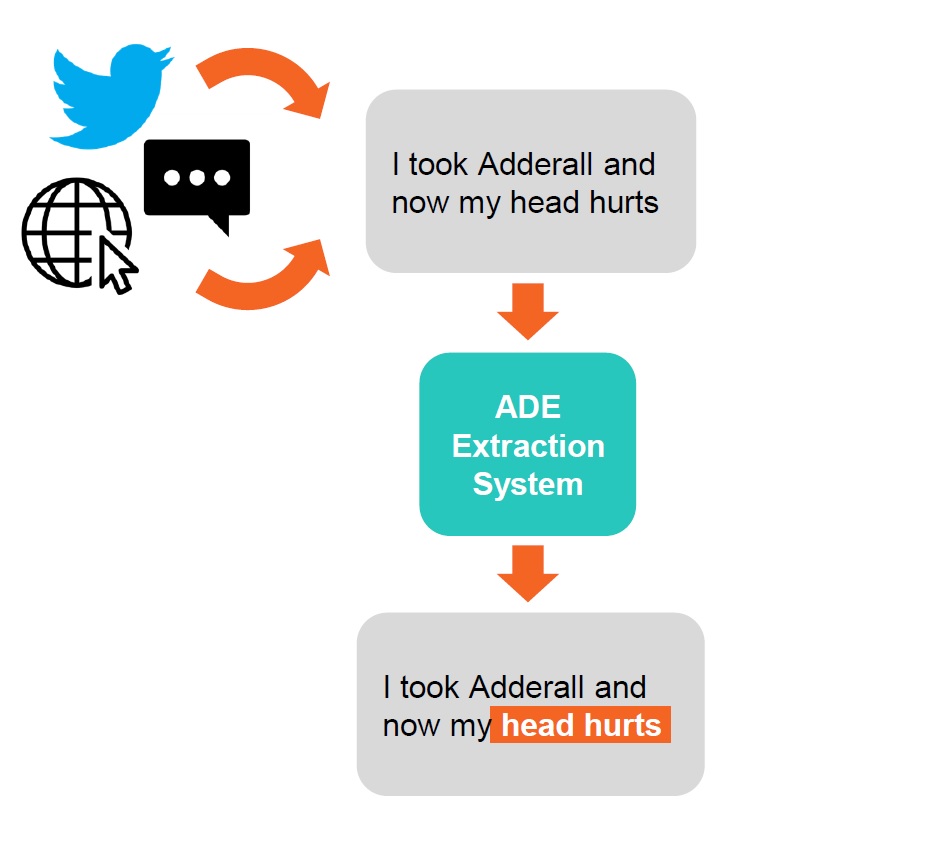
Regulators, such as the Food and Drug Administration (FDA) and the European Medicine Agency (EMA), approve every year dozens of drugs, after verifying their safety and therapeutic effectiveness in clinical trials. Sometimes, however, clinical trials are not sufficient to discover all potential Adverse Drug Events (ADE). Pharmacovigilance, therefore, monitors the drugs in the market to ensure that unexpected effects are immediately identified and actions are taken to minimize their harm.
This process relies on formal reporting methods, such as physician notes. However, a constantly growing number of patients prefers to describe the
side effects on social media platforms, health forums and similar outlets.
Patients have started reporting Adverse Drug Event (ADE) on social media, health forums and similar outlets, often utilizing informal language. Given the need to monitor these sources for pharmacovigilance purposes, systems for the automatic extraction of ADE are becoming an important research topic in the NLP community. Recent shared tasks on the topic of ADE extraction have attracted numerous focused contributions.
Our Latest Achievements
Our research group is working on an architecture for automatic ADE extraction from social media texts, which is able to perform robustly on various different text typologies (from short and noisy tweets to long and formal medical forum posts).
The architecture was benchmarked on one of the most relevant Shared Tasks in this field (Social Media Mining for Health 2019 – SMM4H’19 – Task 2) and is currently on the top of the public leaderboard
(https://competitions.codalab.org/competitions/20798#results).
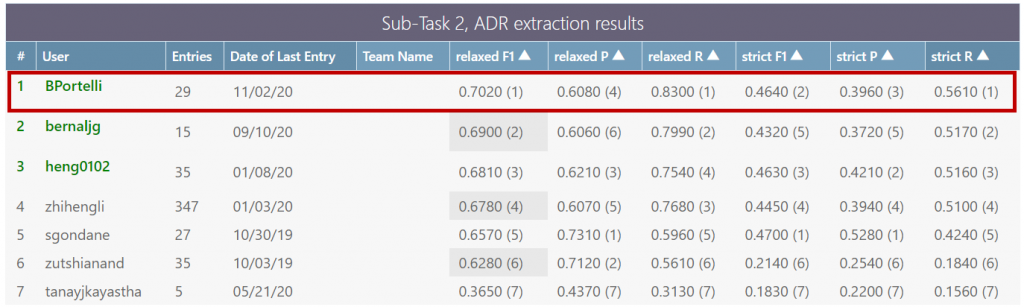
Publications
- Beatrice Portelli, Edoardo Lenzi, Emmanuele Chersoni, Giuseppe Serra and Enrico Santus. (2021).Β BERT Prescriptions to Avoid Unwanted Headaches: A Comparison of Transformer Architectures for Adverse Drug Event Detection.Β Proceedings of the 16th conference of the European Chapter of the Association for Computational Linguistics (EACL 2021). [pdf] [DOI]
- Beatrice Portelli, Daniele Passabì, Edoardo Lenzi, Giuseppe Serra, Enrico Santus and Emmanuele Chersoni. (2021). Improving Adverse Drug Event Extraction with SpanBERT on Different Text Typologies. Proceedings of the 5th International Workshop on Health Intelligence (W3PHIAI 2021). [pdf] [DOI] Award: Best Short Paper
- Simone Scaboro, Beatrice Portelli, Emmanuele Chersoni, Enrico Santus, Giuseppe Serra. NADE: A Benchmark for Robust Adverse Drug Events Extraction in Face of Negations. Proceedings of the 7th Workshop on Noisy User-generated Text (W-NUT) @ EMNLP 2021. [pdf] [DOI]
- Simone Scaboro, Beatrice Portelli, Emmanuele Chersoni, Enrico Santus, Giuseppe Serra. Negation detection for robust Adverse Drug Event extraction from social media texts. Proceedings of the 18th Italian Research Conference on Digital Libraries (IRCDL 2022). [pdf]
Code
Available on GitHub
Research Group
- Beatrice Portelli (AILAB-Udine)
- Edoardo Lenzi (AILAB-Udine)
- Simone Scaboro (AILAB-Udine)
- Giuseppe Serra (AILAB-Udine)
- Emmanuele Chersoni (Hong Kong Polytechnic University)
- Enrico Santus (Bayer)