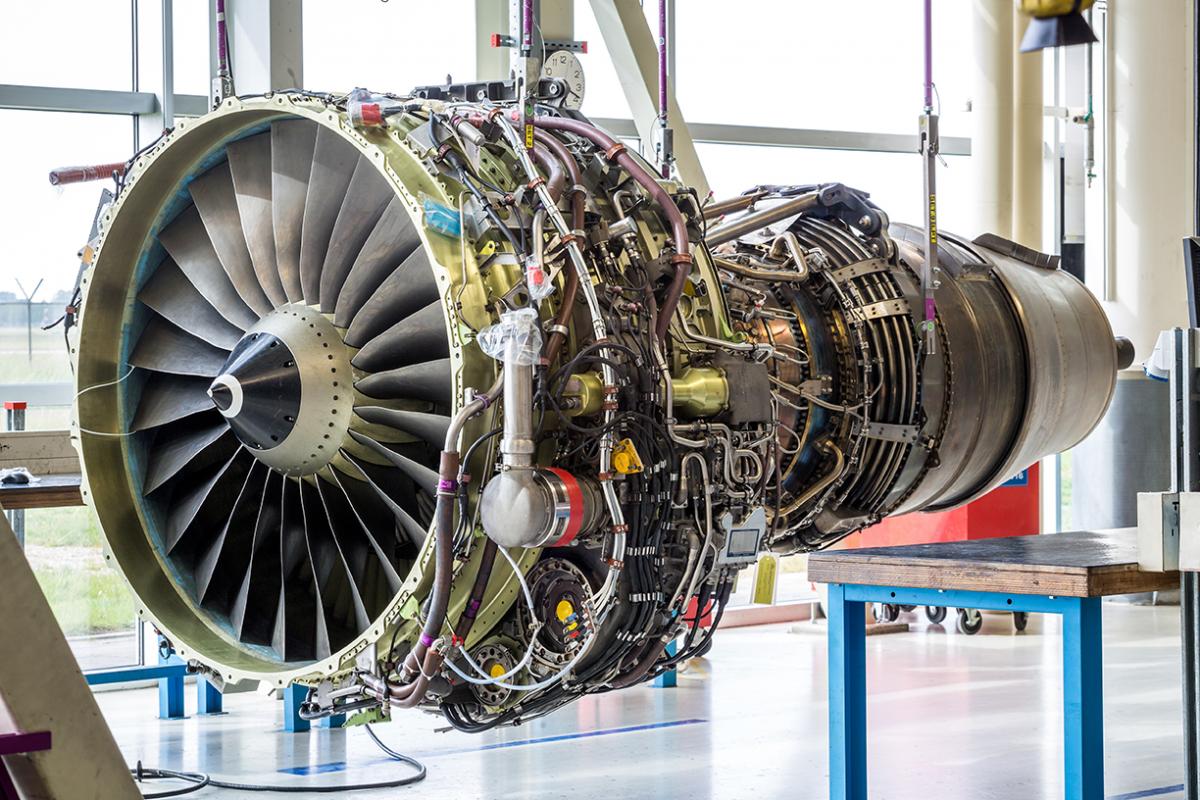
The remaining useful life (RUL) estimation of a component is an interesting problem within the Prognostics and Health Management (PHM) field, which consists in estimating the number of time steps occurring between the current time step and the end of the component life. Being able to reliably estimate this value can lead to an improvement of the maintenance scheduling and a reduction of the costs associated with it.
Data driven approaches are often used in the literature and they are the preferred choice over model-based approaches: in fact, not only they are easier to build, but the data over which they are built can be gathered easily in many industrial applications.
During the last years, neural networks like Long Short-Term Memory (LSTM) and Convolutional Neural Networks (CNN) have found many applications in this area, this because of their suitability to uncover hidden patterns within the sensor data. In recent years a greater availability of high quality sensors and easiness of data gathering gave rise to data-driven models based on deep learning for this task, which has recently seen the introduction of “dual-stream” architectures.
Our research focuses on both single-stream and dual-stream architectures to address the RUL estimation problem through the exploitation of components such as the aforementioned LSTM and CNN, the Neural Turing Machine (NTM) and a Multi-Head Attention (MHA) mechanism.
The NTM is a content-based memory addressing system which gives each of the streams the ability to access to and interact with the memory and acts as a fusion technique. The MHA is an attention mechanism added as a mean for our architecture to identify the existing relations between different sensor data in order to reveal hidden patterns among them. To evaluate the performance of our models, we considered the C-MAPSS dataset, a benchmark dataset published by NASA consisting of several time series related to the life of turbofan engines.
The project is financed by the SMACT Competence Center project “Machine Learning & Data Analytics per l’ottimizzazione di processi” (Machine Learning and Data Analytics for process optimization), a joint project done together with Wärtsilä Italia S.p.A.
Related publications:
- Giovanni D’Agostino, Alex Falcon, Oswald Lanz, Giorgio Brajnik, Carlo Tasso, Giuseppe Serra. Estimating the Remaining Useful Life via Neural Sequence Models: a Comparative Study. 2nd Italian Workshop on Artificial Intelligence and Applications for Business and Industries (AIABI), co-located with AIxIA, 2022. [pdf]
- Alex Falcon, Giovanni D’Agostino, Oswald Lanz, Giorgio Brajnik, Carlo Tasso, Giuseppe Serra. Neural Turing Machines for the Remaining Useful Life estimation problem. Computers in Industry 143, 2022. [pdf] [DOI]
- Alex Falcon, Giovanni D’Agostino, Giuseppe Serra, Giorgio Brajnik, Carlo Tasso. A Dual-Stream architecture based on Neural Turing Machine and Attention for the Remaining Useful Life Estimation problem. Fifth European Conference of the Prognostics and Health Management Society (PHME), 2020. [pdf] [DOI]
- Alex Falcon, Giovanni D’Agostino, Giuseppe Serra, Carlo Tasso, Giorgio Brajnik. A Neural Turing Machine-based approach to Remaining Useful Life Estimation. IEEE International Conference on Prognostics and Health Management (ICPHM), 2020. [pdf] [DOI]
Research Group
- Alex Falcon (AILAB-Udine member)
- Giovanni D’Agostino (AILAB-Udine member)
- Giuseppe Serra (AILAB-Udine member)
- Carlo Tasso (AILAB-Udine member)
- Giorgio Brajnik (Università degli Studi di Udine)